What is MLOps? Benefits and Key Features
MLOps implementation is crucial for businesses to enhance customer experience, provide personalized services, and automate tasks. Check our blog to discover the key features of MLOps and the benefits that it offers to businesses.
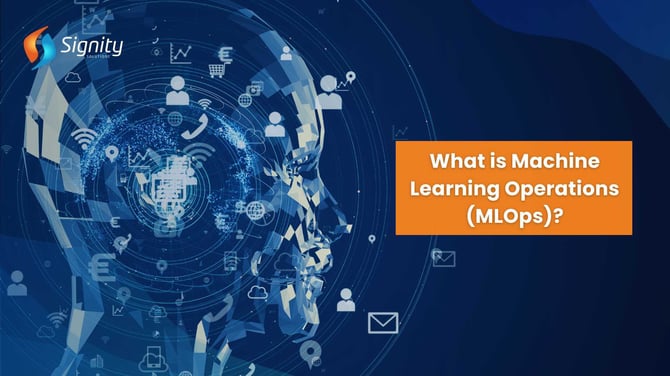
Struggling to keep up with the competition in today's data-driven market? In today’s competitive market, companies need to implement the latest tech stack in order to meet the changing market demands.
MLOps or Machine Learning Operations is the process of combining machine learning, DevOps, and data engineering to streamline the process of building, deploying, and maintaining ML models in production environments.
MLOps solutions aim to improve the collaboration between data scientists, ML engineers, and IT professionals for reliable, scalable, and efficient ML workflows. Whether businesses want to improve model deployment, enhance collaboration, or reduce operational costs, MLOps implementation is the perfect solution.
Partnering with leading MLOps services, entrepreneurs can leverage the power of technology for better ROI.
Key Takeaways
- MLOps automates the entire ML process, ensuring efficiency and reliability.
- MLOps platform optimizes scalability and resources, reducing costs and maximizing ROI.
- It provides real-time insights, enhancing customer experiences and business outcomes.
- MLOps ensures model transparency and reliability, helping manage risks and maintain compliance.
Key Features of MLOps Solutions
Want to know what are the key features of MLOps? Here are the essential features of MLOps solutions that make it a must for businesses across all industries:
-
End-to-End Automation
MLOps systems provide a complete solution for automating every stage of the ML process. MLOps tech eliminates manual involvement and potential errors at each stage by combining data collection, preparation, model training, deployment, and monitoring into a single process.
Without the need for continual human supervision, this end-to-end automation guarantees that machine learning models are created, implemented and maintained effectively. These platforms make use of cutting-edge technologies like cloud infrastructure, CI/CD pipelines, and containerization.
-
Scalability Optimization
MLOps platforms are excellent at maximizing machine learning models' performance, scalability, and deployment in a variety of operating contexts. It gives businesses the opportunity to grow their AI initiatives effectively without sacrificing model performance or reliability.
MLOps platforms ensure that machine learning models can be easily scaled up or down to meet changing demands while maintaining consistent and reliable operation across a variety of computing environments.
Businesses can swiftly implement and modify their AI-powered products to match changing market demands and maintain an advantage over rivals thanks to this scalability optimization.
-
Standardization
Another key feature of the MLOps platform is that it offers a thorough coordination of workflows for ML, standardizing and automating the many processes involved. It comprises tools that manage various phases of the machine learning lifecycle, from data preparation to monitoring.
It eliminates manual errors and facilitates smooth collaboration between data scientists, machine learning engineers, and IT specialists.
-
Integration with DevOps and CI/CD
MLOps can be easily integrated with DevOps and CI/CD. The integration facilitates the development, deployment, and maintenance of machine learning models by allowing data science and engineering teams to work together harmoniously through the use of common tools, procedures, and workflows.
This feature allows businesses to automate the model deployment process, apply stringent quality checks, and guarantee the dependability and consistency of their AI-powered solutions by integrating MLOps into the CI/CD pipeline.
-
Democratization
A noteworthy feature called "MLOps democratization" aims to increase machine learning accessibility for a wider group of stakeholders and users. MLOps platforms are removing entry barriers for non-experts by offering low-code/no-code MLOps solutions and user-friendly interfaces, making it simple for users to design, deploy, and manage machine learning models.
Without requiring in-depth technical knowledge, this democratization of MLOps enables business analysts, domain experts, and even citizen data scientists to actively engage in the machine learning process.
These are some of the features that make MLOps an essential part of each business. The next section talks about the benefits of the MLOps tool in detail.
Don't Get Left Behind: MLOps is the Future of AI
Stay ahead of the competition with a well-defined MLOps strategy and unlock the full potential of your AI investments.
Benefits of MLOps Solutions for Businesses
Did you know that the global MLOps market size is expected to reach $13,321.8 million by 2030? It is an essential function of ML engineering and holds various benefits for businesses. Let’s check these one by one.
-
Faster Time to Market
Businesses can considerably speed up the development and deployment of new machine learning-powered products and services with the efficiency improvements generated by MLOps services. MLOps helps organizations shorten the time it takes to move from model development to production deployment by automating repetitive operations, facilitating collaboration, and enhancing the overall stability and consistency of the machine learning lifecycle.
Compared to traditional, manual machine learning workflows, this enables organizations to rapidly iterate on new ML-driven technologies and get them into the hands of customers considerably faster.
-
Cost Efficiency
Another major benefit of MLOps implementation is a reduction in costs. MLOps lowers the costs and human labor involved in creating, training, and deploying machine learning models by automating the provisioning and control of infrastructure resources.
“One real example of this can be seen when PadSquad, a mobile software company, wanted to lower its media costs. With the help of the Iguazio data science platform that is built for production and real-time machine learning applications, PadSquad was able to reduce media costs incurred by its customers.”
MLOps enables significant cost cutting, particularly for enterprises with extensive machine learning implementations, enabling them to optimize the ROI in AI and data science projects.
-
Enhanced Model Reliability and Performance
Businesses can enhance the performance and dependability of their machine learning models with the reliable implementation of MLOps. Automated testing and continuous monitoring ensure that the models consistently meet the necessary performance requirements, providing a sense of security and assurance in business operations. This is achieved by regularly assessing the models' precision, resilience, and adaptability to changes in the data and user behavior.
Further, they can trust the results of their machine learning systems and make better informed, data-driven decisions that propel company success by proactively correcting any problems or drifts in model performance.
-
Real-Time Insights
MLOps gives companies the real-time insights and data-driven capabilities they need to improve customer experiences. MLOps helps organizations to quickly adapt their machine learning-powered applications and services to changing customer needs through scalable infrastructure management, automated model deployment, seamless data ingestion and processing, centralized model governance, and continuous model monitoring and optimization.
“Netflix utilizes MLOps to deliver the right recommendations to their users. The company does so by constantly monitoring data to ensure users are provided with suggested content they will like. Therefore, these ML models enable Netflix to get real-time insights to lend the perfect services.”
In the end, implementing MLOps leads to increased customer happiness, loyalty, and economic success since it enables entrepreneurs to provide individualized, pertinent, and dependable experiences that satisfy changing user expectations.
-
Improved Decision-Making
Another major benefit of MLOps development is enhanced decision-making at every level. By leveraging ML models that are regularly updated with the latest tech MLOps practices, businesses can gain a better understanding of their operations, customer behavior, and market trends.
Here is another great example of a leading brand that enhanced its performance with key components of MLOps.
“Starbucks faced a problem in providing personalized recommendations to their customers. With its AI platform, Deep Brew, Starbucks was able to provide personalization along with assisting workers with administrative activities in the store. Deep Brew was this company's first invasion into ML, and it built a team of data scientists to prototype the recommendation engine and other models.”
Hence, this MLOps implementation helped the brand enhance its decision-making. Accurate MLOps tools serve as a base for building effective strategies and improving business outcomes.
-
Automated Monitoring and Maintenance
Another major advantage of machine learning operations is its ability to save time for monitoring and maintenance. It eliminates the need for manual involvement because automated methods for model retraining and fine-tuning keep the models current with the most recent data.
This proactive approach to model maintenance guarantees that businesses can depend on reliable, high-quality insights to inform their decisions while reducing the risks of model degradation. MLOps helps companies to effectively grow their machine learning efforts while minimizing the resources needed for continuous model administration by automating these crucial operations.
-
Risk Management and Compliance
Businesses can gain significant benefits from MLOps tools and procedures in terms of risk management and compliance. Further, companies can guarantee the transparency and audibility of their machine-learning systems by monitoring the history of their data and preserving version control over their models.
Moreover, businesses can reduce the risks associated with obsolete or defective models by proactively having the capacity to promptly detect and resolve any faults or drift and monitor the operation of the model. This proactive approach ensures businesses are always prepared for any potential issues.
“Moody's, a financial services company, is one of the greatest examples that recorded continuous improvement with the MLOps lifecycle. With the help of the MLOps implementation process, Moody's reduced the risk of errors, making it much faster to get improved models into production.”
Establishing strong MLOps procedures enables businesses to show stakeholders and regulatory agencies that their data and models are reliable.
Empower Your Business with Cutting-Edge MLOps Services
Streamline your workflow, reduce costs, gain real-time insights, and boost business growth with our top-notch MLOps services.
Grow Your Business With Our MLOps Services
Looking for leading MLOps services? Partner with Signity Solutions and work with the right MLOps tools. Our MLOps services include:
- Automated ML Workflows
- Model Version Control
- CI/CD for ML
- Model Deployment Automation
- A/B Testing for ML Models
- Model Monitoring & Explainability (MLOps)
- MLOps Consulting
- Security & Governance for MLOps
Working with our ML model will help you improve model performance, reduce costs, enhance scalability, increase efficiency, and get expert guidance whenever needed.
Hire a dedicated team of MLOps engineers today to grow your business with the latest MLOps tools.
Frequently Asked Questions
Have a question in mind? We are here to answer. If you don’t see your question here, drop us a line at our contact page.
What are MLOps benefits?
Several benefits of MLOps include faster time to market, enhanced customer experience, personalization, and cost efficiency.
What are the key features of MLOps?
Key features of machine learning operations include integration with DevOps and CI/CD, automation, scalability, and democratization.
What is meant by MLOps?
MLOps is the practice of combining machine learning and operations to streamline the deployment, monitoring, and management of machine learning models in production.
What are the key outcomes of MLOps?
The key outcomes of MLOps are not just theoretical, but they lead to improved model deployment, monitoring, and management in production environments. This results in more efficient and reliable machine learning operations.
How does MLOps differ from traditional DevOps?
MLOps incorporates specific machine learning development processes, such as data versioning and model performance monitoring, while DevOps focuses on streamlining software development and deployment processes.