Enterprise RAG Security: Key Safeguards for Business Success
Explore the critical security considerations for RAG deployments, including data integrity, privacy concerns, system availability, and effective resource management. Discover how addressing these key considerations enables scalable AI solutions for enterprises. Read the full blog for expert insights!
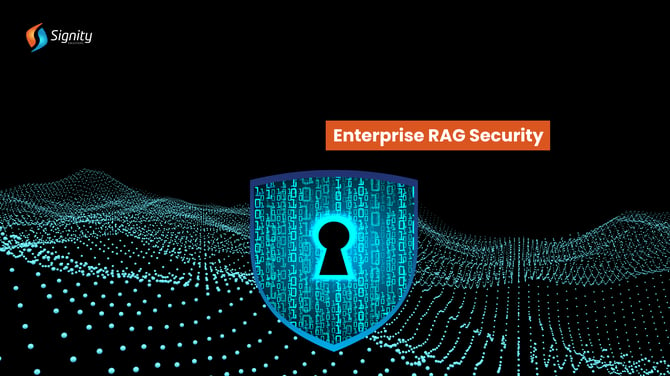
Artificial intelligence is no longer a futuristic technology. It's a rapidly evolving field. One promising area of AI innovation is Retrieval Augmented Generation (RAG).
Imagine your AI assistant not just relying on its pre-trained knowledge. But also intelligently accessing and incorporating information from your company's specific documents, databases, and knowledge repositories in real-time.
That's what Retrieval Augmented Generation solutions bring to you.
Since RAG interacts directly with your sensitive business information, it also poses security risks, including data exposure and unauthorized access to sensitive data. So, if you do not have robust security measures in place, your business may face potential risks and even damage to its reputation.
Therefore, security cannot be an afterthought in your RAG deployment strategy. In this blog, we will discuss important security considerations for RAG systems. We will explore the potential security attacks and best practices to avoid them.


-
To protect sensitive business and customer information, use strict privacy controls from ingestion to storage in vector databases. This helps protect against regulatory risks.
-
Always encrypt data, both when it is stored and when it is being transferred. This ensures the data remains unreadable even if someone gains unauthorized access.
-
Improve security for RAG deployments by addressing vulnerabilities such as unauthorized access, data leaks, and system downtime at every level.
-
Regularly check security and monitor the data storage for any signs of breaches or vulnerabilities. Quick detection and response can limit potential damage.
RAG Architecture and Associated Security Risks
RAG is a technique used to improve the performance of Large Language Models (LLMs) by providing them with access to external knowledge bases.
But these RAG-based generative AI solutions also pose significant security risks. These include data poisoning, malicious data embedding inversion, prompt injection, and data leakage. All of these risks can harm the confidentiality, integrity, and availability of sensitive data.
The RAG architecture consists of a retrieval system, a vector database, and a generation stage, each of which introduces unique security risks. Implementing RAG systems with a focus on security and privacy concerns is vital.
Before moving on to the best practices, take a look at the key risks associated with each stage.
Security Risks at Vector Databases
Vector databases index, store, and retrieve vector embeddings that are converted from unstructured data. Vectors are repositories of valuable information in RAG architecture. They store sensitive data and can be used to gain access to confidential information. These can also be prime targets for unauthorized access and data breaches.
Engineering teams face significant security concerns when handling private data in vector databases, particularly due to potential vulnerabilities in immature systems. Embeddings, which are mathematical representations of data, can inadvertently contain sensitive and personally identifiable information (PII).
So, businesses must understand the associated security risks with this stage. Let us begin.
1. Data Integrity Threats
Vector databases store embeddings that form the foundation of your RAG system’s retrieval capabilities. But, they rely on accurate and trustworthy embeddings to deliver relevant responses.
However, without proper protection measures, these databases can be tampered with. This tampering can be either unintentional or through malicious actions.
When embeddings, which are mathematical representations of data, are tampered with or corrupted, your RAG system may retrieve incorrect information. This can lead to inaccurate outputs and compromised decision-making for end-users.
That is why ensuring the integrity of data stored in vector databases is essential. Compromised data integrity in these databases poses significant business risks.
2. Data Privacy Concerns
Vector databases often store embeddings that come from sensitive business documents, customer data, and proprietary information. Embeddings, which are mathematical representations of data, can inadvertently contain sensitive information that may pose privacy risks.
Attackers can retrieve the original data through inversion methods from vector databases. Due to this, vector databases can be vulnerable to data leaks or unauthorized access.
3. System Availability Issues
RAG deployments rely on the availability of vector databases. If the system goes down, even for a short time, it can disrupt the entire AI experience. This affects your ability to retrieve context for AI responses and can lead to lower user satisfaction and interruptions in business operations.
4. Data Minimization
In the rush to provide RAG systems with as much context as possible, businesses may end up storing more data than they need. This violates the principle of data minimization, which is a core requirement of many data protection laws.
5. Data Anonymization
Even at the vector level, we need to protect sensitive information. Anonymizing customer or internal data is essential before storing it in vector form. If we include unmasked names, identifiers, or business secrets, we risk privacy violations if that data is ever exposed or accessed improperly.
6. Proliferation of Private data
A major concern with RAG is the use of vector databases, which store embeddings derived from private data. These embeddings can sometimes be reversed through inversion attacks that expose sensitive information. As vector databases are relatively new, their security features are not yet fully developed, which can lead to potential bugs and vulnerabilities.
Don’t let Security Risks stall your AI Innovation Journey
Partner with Signity AI development experts to deploy safe, compliant, and powerful RAG systems.
Security Risks at Retrieval Stage
The retrieval stage is a crucial step, and it is important to control who can access the knowledge base through queries. Validating user queries is essential to ensure data security and prevent unauthorized access. User queries play a significant role in initiating the retrieval process and ensuring data security through validation and access controls.
Security measures in the retrieval processes, including query validation, access control, and data integrity, are vital to prevent data leakage and ensure secure transmission of data. Robust data protection through strong encryption methods is critical. Capturing semantic meaning during the pre-processing stage in RAG systems is important.
Here are some risks associated with this stage:
Prompt Injection Attacks
A prompt injection occurs when someone inserts hidden instructions into a system, thereby overriding its original settings and potentially compromising its security. Implementing a validation process for user queries is crucial to prevent malicious input from exploiting the system, thus enhancing overall security and integrity.
In contrast, prompt leaking involves sharing sensitive information with individuals who should not have access to it, as illustrated below.
Attackers often trick applications by putting misleading text in user questions. This enables them to perform unwanted actions or disclose confidential information.
For example, a data analyst with limited access to patient records uses a chatbot to get approved summaries. An intruder can include a secret command in a normal question. This can cause the chatbot to disregard its privacy rules and expose personal health records that should remain confidential.
PII Exposure and Privacy Leaks
RAG systems can inadvertently expose personally identifiable information (PII) if proper precautions are not taken. When retrieving sensitive data from databases or handling private details submitted by users, the system might unintentionally reveal confidential information.
For example, an employee might query an internal RAG system and receive another employee’s personal or salary information by mistake.
Knowledge and Data Poisoning
RAG relies on external data sources. This puts it at risk of attacks that give false or harmful information, known as data or knowledge poisoning. Specifically, poisoned data can lead to poor performance in large language models (LLMs) by compromising the integrity of the information provided to users.
This is particularly concerning in retrieval-augmented generation (RAG) workflows, where sources that may contain poisoned data can significantly impact the outputs of AI.
An attacker could change internal databases or upload misleading documents. This can cause the AI to produce responses that are harmful or incorrect. Even false content from outside sources can deceive the system, while leading to incorrect decisions or the spread of misinformation. Protecting against poisoned data is also crucial to maintain the integrity of the information provided to users.
Dynamic Data Retrieval
Integrating with external knowledge sources can put sensitive data at risk if the method of accessing that information is not secure. It is crucial to implement robust security measures in the retrieval processes, including query validation and access control. Ensuring data integrity during information retrieval involves differentiating user access levels to prevent data leakage. Additionally, secure transmission of data through encryption and updated protocols, along with regular auditing, helps identify potential security threats.
LLM log leaks
User prompts, especially when combined with additional data, can expose sensitive information. This data may pass through systems that could be hacked or contain bugs, which often log prompts and responses.
For instance, OpenAI has faced issues like account takeovers and data leaks. Although some customers can control how long records are retained, using private data with third-party models remains a risk. Unauthorized access to sensitive content within RAG workflows can result in significant privacy breaches and legal consequences. Even self-hosted language models still carry potential threats.
Security Risks at the Generation Stage
One of the primary concerns regarding RAG workflows is the risk of unauthorized access to sensitive information. The generation stage of a RAG flow is vulnerable to security threats such as prompt injections.
Just like the other stages, the Generation stage also presents a set of risks while generating output. Have a look at the generation stage-associated risks below:
Bias and Offensive Content
If the training data includes bias or offensive content, the language model may generate biased or offensive responses. This can create legal risks, especially in production settings.
Legal liabilities associated with generating biased or offensive content and potential data privacy violations can lead to reputational damage and legal consequences. This underscores the importance of adhering to content standards and implementing security measures to prevent unauthorized data exposure.
Output Manipulation
Attackers can change the output of a large language model (LLM) by adjusting the input queries. This could result in the dissemination of harmful or misleading content. The risk of generating harmful outputs is particularly concerning when attackers manipulate inputs or databases. This is especially worrying in situations where people trust the outputs without checking them.
Proactively manage AI risks with secure RAG solutions
Protect your business from data leaks, misinformation, and operational vulnerabilities.
Best Practices to Secure RAG Deployment
RAG applications consist of several layers that work together. And each one requires addressing the security concerns beforehand. That is why it is essential to have security best practices in place. Implementing RAG systems with a focus on security and privacy concerns is crucial.
This includes careful selection of models and secure data storage to mitigate risks such as data poisoning and leakage of sensitive information. Addressing these vulnerabilities requires a comprehensive strategy that includes robust encryption and continuous oversight.
Here are some key best practices to secure RAG applications effectively:
Use Guardrails in Prompt Engineering
Guardrails are predefined rules or constraints that limit the behavior and output of AI systems. Use prompt engineering techniques to create clear boundaries for the model's responses. This involves using specific phrases, such as <question> and <answer>, to guide the model in its output.
By setting these guidelines, you can reduce the chances of receiving unwanted replies. Adding these guardrails will help create strict responses, keeping unwanted behaviors in check and preventing outside prompts from affecting the output.
Use Privacy-Preserving Techniques
- Differential Privacy: This method adds small amounts of random noise to data. It helps protect individual identities while keeping the usefulness of the data. It also meets rules like GDPR.
- Federated Learning: This approach allows training without moving sensitive data from its original location. While effective, it can be complicated due to communication issues and synchronization challenges.
Use Salted Tags to Prevent Tag Spoofing
Randomly generated tags, such as <secure-12345>, are added to XML-like tags in prompts. This makes it difficult for attackers to guess the right tags. This also lowers the risk of unwanted instructions being inserted under a false cover by using trusted inputs.
Implement Zero-Trust Architecture
A zero-trust framework requires strict access controls and checks all interactions within the system. This helps prevent unauthorized access to data and improves security in environments with multiple users.
Zero-trust architecture requires constant verification at every level. It views each part of the data flow as untrusted. It uses identity-based access control to ensure that only authorized people can handle data ingestion, embedding, and retrieval.
Additionally, micro-segmentation divides the pipeline into separate zones, which limits the damage if an attacker breaches the system. In a RAG application, each stage requires re-authentication and permission checks. This helps reduce the risk of lateral movement or data leakage.
Also Read: Enterprise RAG: Why Retrieval-Augmented Generation is Future of AI?
Incorporate Access Control
Establish strict access rules for each part of the RAG system, including retrieval, generation, and storage, to ensure secure and controlled access. Use Role-Based Access Control (RBAC) to ensure that only authorized users can access or modify sensitive data. For instance, limit access to sensitive retrieval sources to stop unauthorized data requests.
Use Multi-Factor Authentication (MFA)
Secure sensitive components, particularly those involving PII(Personally Identifiable Information). Implement multi-factor authentication to access the retriever and generator systems.
Implement Output Filtering and Sanitization
Use response filters to detect and mask sensitive information or PII before it reaches end-users. Filtering could include redacting or anonymizing PII using natural language processing tools, which can be customized to identify and sanitize specific data types.
Incorporate Data Encryption Mechanisms
Symmetric algorithms, like the Advanced Encryption Standard (AES), protect stored data. While the asymmetric methods, such as Rivest-Shamir-Adleman (RSA), secure information that moves through query pipelines. These methods lower the risk of exposing sensitive data or user inputs during transmission.
Application-layer encryption adds another layer of protection. This ensures that even if someone breaches the storage environment, they cannot access the decrypted content without the right keys.
Also, use encryption methods like homomorphic encryption and TLS to protect data when stored and shared. These techniques help shield against unauthorized access and ensure compliance with data security standards.
- You can set up data validation checks on input and output to ensure data is accurate and to block harmful content from external sources.
- Secure your system’s API endpoints with strong authentication methods, such as OAuth2.
- Use end-to-end encryption for all communication between the RAG system and other sources.
- You must utilize continuous monitoring tools to identify unusual activity or breaches quickly. This enables a fast response to incidents.
- You can also include external threat intelligence feeds to help identify possible weaknesses and attack methods in real-time.
End-to-End Security Controls
End-to-end security controls are essential for ensuring the security and integrity of RAG systems. These controls include implementing robust security measures, such as access controls, data encryption, and output validation, to protect the RAG system from malicious actors.
Moreover, RAG systems must also implement incident response plans to respond to security incidents and minimize their impact quickly. By implementing end-to-end security controls, RAG systems can ensure that they are properly secured and that sensitive data is protected.
Conclusion
Securing RAG applications requires a structured approach to prevent data breaches, unauthorized access, and prompt injection attacks.
By utilizing robust, prompt engineering, safe data storage, and real-time monitoring systems, organizations can mitigate specific risks related to RAG.
As AI systems like RAG become more advanced, it is essential to adopt these safety measures to protect against growing security threats. We hope this blog provided you with up-to-date information on the best security practices and possible security vulnerabilities of RAG systems.
Planning to implement RAG systems with the best security measures. At Signity Solutions, we design and build secure and scalable RAG architectures tailored to meet your business needs. Our expertise lies in AI development and protecting your systems from potential risks while maximizing business value.
Frequently Asked Questions
Have a question in mind? We are here to answer. If you don’t see your question here, drop us a line at our contact page.
What does RAG stand for?
RAG stands for Retrieval-Augmented Generation. It is a system that enhances large language models (LLMs) by allowing them to draw information from an external knowledge base. This helps them give more accurate, relevant, and current answers.
What are the Security concerns of RAG?
Retrieval-augmented generation (RAG) has several security risks, including data injection, unauthorized access, information leaks, prompt injection attacks, and flaws in retrieval systems. Malicious users can add harmful data, and prompt injection attacks can compromise the operation of RAG systems.
What is RAG security?
RAG security aims to protect the Retrieval-Augmented Generation (RAG) system and its data from unauthorized access and leaks. This includes ensuring the accuracy of the documents we retrieve, controlling access to the generated content, and mitigating risks associated with using external data sources that incorporate large language models.
What are the Privacy Issues with RAG?
Privacy issues with RAG arise during data retrieval. If the knowledge base contains sensitive or personal information, this data could be exposed or misused. To address this, it’s crucial to implement data anonymization, access controls, and comply with privacy regulations when using RAG.