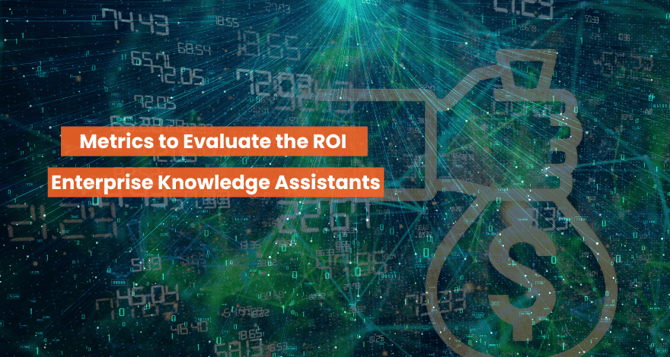
Information is everywhere in modern enterprises, but insights are very rare. Different teams need to hop to various tools, dig through the dashboards, and connect to coworkers to find answers that already exist in the system. This growing gap between knowledge and access is not just causing inconvenience but also distractions in focusing on work, clarity, and ideal decision-making.
Enterprise Knowledge Assistants are AI-powered copilots embedded into everyday workflows. These assistants aren't just searching with better manners; they understand intent, retrieve contextually relevant information, and deliver it in real-time.
Whether it's guiding a customer support representative through a complex policy or helping a new employee ramp up without handholding, Enterprise Knowledge Assistants are quietly transforming how organizations operate.
Frequently Asked Questions
Have a question in mind? We are here to answer. If you don’t see your question here, drop us a line at our contact page.
It's more than simply a trend, either. Enterprise Knowledge Assistants equipped with Retrieval Augmented Generation are being quickly embraced by leaders in various industries, including healthcare, technology, manufacturing, and finance, as they recognize that they can facilitate smooth communication, accelerate data-driven decision-making, and produce measurable productivity improvements.
Despite all the hype, one important question still needs to be answered: Are they truly delivering value to the enterprise?
In this blog, we'll go through five metrics that matter, real indicators that help you evaluate the ROI of your Enterprise Knowledge Assistant, not just in theory but in everyday impact.


- Implementing a knowledge assistant is only half the equation; tracking its real-world impact through structured metrics is what turns it into a strategic asset.
- With the right metrics, like search time reduction, onboarding efficiency, and training cost cuts, you can quantify direct operational gains.
- Faster, more accurate responses through EKAs improve CSAT, reduce churn, and enable scalable service delivery, driving both retention and growth.
- By equipping support teams with accurate, grounded responses, Enterprise RAG assistants improve resolution speed and reduce escalations.
Why ROI Measurement Isn't Optional Anymore?
Investing in Enterprise Knowledge Assistants isn't just about adopting the latest AI trend; it's about solving real problems. But like any strategic investment, simply implementing an EKA isn't enough.
You need to know:
Is it working? Is it worth it? And where do we go from here?
That's where measuring ROI becomes essential.
Quantifying the return on your EKA helps you answer the tough questions:
- Is it genuinely reducing time spent on repetitive queries?
- Are employees actually relying on it or avoiding it altogether?
- Is it impacting business outcomes like faster resolutions, better decisions, and smoother onboarding?
It's simple to slip into the trap of attractive-looking but incomplete success adoption dashboards in the absence of precise measurements.
A solid ROI framework not only justifies the investment to stakeholders but also guides future enhancements, helping you fine-tune models, expand use cases, and deliver even greater value across teams.
Metrics for Assessing Enterprise Knowledge Assistant ROI
Here's a comprehensive list of metrics for assessing the ROI of Enterprise Knowledge Assistants, structured across technical, user-centric, operational, and strategic dimensions. These are especially relevant for RAG-powered systems and are aligned with best practices in enterprise AI evaluation.
1. Search Accuracy and Relevance
This metric evaluates how precisely the Enterprise Knowledge Assistant (EKA) retrieves and presents relevant, correct, and context-aware information from your internal data sources, whether that's documents, wikis, support tickets, or product manuals.
According to a McKinsey & Company survey, employees spend 1.8 hours a day on average looking for relevant knowledge, according to recent data.
Trust starts with accuracy. If the assistant delivers outdated or irrelevant answers, users will stop relying on it, leading to confusion, wasted time, and workarounds.
You need to track both system and user-centered metrics:
Trust starts with accuracy. If the assistant delivers outdated or irrelevant answers, users will stop relying on it, leading to confusion, wasted time, and workarounds.
You need to track both system and user-centered metrics:
- Precision – % of retrieved answers that were actually relevant
- Recall – % of all relevant answers the assistant managed to find
- F1 Score – Balance between precision and recall
- NDCG – Evaluates the ranking quality of results shown to users
Case Study
A global enterprise software company introduced a RAG-powered Knowledge Assistant to help internal teams access critical documents across platforms like Confluence, Google Drive, and SharePoint. While adoption was quick, trust lagged behind.
Users reported unclear or outdated answers, and support agents still had to cross-check responses manually, reducing the productivity gains the assistant was meant to deliver.
Optimization Steps:
- Built a ground truth dataset from 500 real queries.
- Switched to a hybrid retriever (BM25 + domain-trained dense model).
- A reranking layer was added, prioritizing recent, role-relevant content.
- Enabled user feedback with thumbs-up/down and optional comments.
Within 60 days, measurable improvements were evident. Precision rose from 0.69 to 0.92, recall improved to 0.87, and the F1 score climbed to 0.89. Users reported far higher satisfaction, with positive feedback increasing threefold.
Operationally, the support team saw a 22% drop in escalations and resolved tickets 40% faster. Even onboarding new employees became easier, with first-week self-service success rates doubling.
This transformation showed that improving search accuracy wasn't just a technical win. It had a direct and sustained impact on efficiency, trust, and overall ROI.
Turn Your Enterprise Data into Actionable Insights with RAG
Connect your internal documents to a smart assistant that actually knows where everything is.
2. Time Saved in Information Retrieval
This metric measures how much less time employees spend searching for information after your Enterprise Knowledge Assistant has been deployed. It focuses on reducing one of the biggest hidden productivity drains in modern workplaces: context switching and knowledge hunt.
By minimizing the time it takes to find accurate, relevant information, EKAs empower teams to stay focused, move faster, and make decisions with confidence. In short, this is one of the most direct ROI factors for any knowledge assistant.
How to Measure
- Before vs. After Comparison: Use surveys or productivity tools to benchmark average search time pre- and post-EKA.
- Query Resolution Analytics: Track how long it takes the EKA to return usable answers.
- Tool Interaction Logs: Measure time from query to action within integrated apps like Slack or intranet portals.
- Role-Based Breakdown: Analyze time saved by function to understand department-level impact.
Case Study
At a global IT services firm with over 300 support agents, handling complex client issues required frequent lookups across multiple internal systems, Confluence pages, old email threads, SOP documents, and chat histories.
On average, agents spent 8–10 minutes per ticket just trying to locate the right process flow, escalation matrix, or policy guideline before they could even begin resolving the issue.
The company implemented a RAG-powered Enterprise Knowledge Assistant, which was integrated directly into their Zendesk and internal chat systems. The assistant was trained on product documentation, internal SOPs, known issue resolutions, and historical tickets.
It used natural language queries to surface relevant snippets instantly and pointed agents directly to the source documents, complete with confidence scores and citations.
Within the first month:
- The average search time per ticket dropped from 9 minutes to just 47 seconds.
- Query logs showed that over 85% of agents began relying on the assistant for ticket triaging and response crafting.
- The assistant handled 22,000+ queries, with a 92% resolution rate without further escalation.
Over a quarter, this translated into:
- 2,200+ agent hours saved
- A 17% increase in the first-contact resolution rate
- A 12% drop in customer wait time
- A measurable 3-point increase in CSAT scores
Additionally, the knowledge assistant's usage data helped the knowledge management team identify documentation gaps, which led to the creation of 50+ new SOPs and guides, further reducing friction in future ticket resolutions.
3. Reduction in Training and Onboarding Costs
This metric measures how much your Enterprise Knowledge Assistant (EKA) reduces the time, effort, and cost associated with onboarding new employees. It reflects the assistant's ability to provide on-demand answers, contextual guidance, and real-time learning without requiring constant intervention from managers or HR.
Traditional onboarding is time-consuming, expensive, and often inconsistent. New hires spend days navigating learning portals, scheduling training sessions, or relying on colleagues for tribal knowledge. Every delay in onboarding translates to lost productivity, increased dependency, and higher cost-per-hire.
An EKA acts as a self-service onboarding companion, guiding employees through policies, tools, workflows, and FAQs, helping them ramp up faster and with fewer disruptions.
How to Measure
- Training Hours Reduction: Compare average hours spent in formal training sessions before and after EKA deployment.
- Training Cost Reduction: Track reductions in costs related to trainers, materials, and scheduled sessions.
- Time-to-Productivity: Measure how long it takes for new employees to start contributing meaningfully.
- Helpdesk Dependency: Track the drop in internal support tickets from new hires for basic queries.
Case Study
A mid-sized SaaS company onboarding 25–30 new employees each quarter found that each new hire required 15–18 hours of scheduled training and frequent 1:1 sessions with managers to understand tools, processes, and internal knowledge.
After integrating a domain-specific EKA trained on company documents, playbooks, policies, and past employee FAQs:
- Formal training hours dropped by 40%
- The average time-to-productivity went from 3.5 weeks to 2.1 weeks.
- Internal support tickets from new hires fell by 60%, freeing up HR and IT bandwidth.
Over two quarters, this translated into great cost savings on training resources while improving new hire satisfaction and early performance metrics.
4. Customer Satisfaction and Service Quality
Using metrics like Customer Satisfaction, Net Promoter Score, and average resolution time, this metric examines how a knowledge assistant enhances the overall customer experience.
Every support ticket is the result of a consumer who wants accurate, timely, and non-distracted responses. Agents respond more fluidly and confidently when they are equipped with the appropriate knowledge at the proper moment. Clients return when they sense clarity and concern.
A positive experience does more than solve an issue; it increases brand strength, fosters customer loyalty, and even creates opportunities for future sales.
Customer support knowledge assistants excel in this situation. They assist your team in creating moments that feel better because they are better by cutting down on back and forth, doing away with guesswork, and providing solutions immediately.
How to Measure
- Track CSAT and NPS Scores: Watch for improvements in post-interaction surveys and overall sentiment.
- Measure Resolution Time: Has the average time to solve a customer query dropped?
- Monitor First Contact Resolution: Are more issues being solved on the first try?
- Run A/B Tests: Compare outcomes between teams using the assistant and those who aren't.
RAG-Specific Considerations
RAG-based assistants don't just give fast answers; they provide accurate, grounded, and contextual answers. This is especially important for complex queries where outdated or vague responses would normally frustrate a customer. When responses are backed by real documentation, trust naturally follows.
Case Study
At a growing e-commerce company, customer support agents were juggling multiple tools to answer basic questions like, "Can I return a sale item?" or "Where's my package?" It led to long wait times, slower resolutions, and, understandably, frustrated customers.
After rolling out a RAG-powered knowledge assistant directly inside their support dashboard:
- Agents were able to respond 28% faster since the assistant pulled up the exact policy or order info in seconds.
- CSAT jumped by 11 points in just over a month.
- The number of issues resolved in the first response increased by 22%, with clear, confident answers.
- A/B testing revealed that EKA-assisted agents closed more tickets per hour, with fewer escalations and better consistency.
The assistant was also used to power the company's help center, helping customers self-resolve common issues, cutting total ticket volume by nearly one-fifth.
5. Financial Impact
This metric captures the tangible financial outcomes driven by a knowledge assistant, both in terms of operational cost reductions and revenue gains.
A Market Logic analysis on hyperscaling insights states that cost savings can improve by 15% to 20% just by using consumer knowledge effectively. At the end of the day, every AI investment, no matter how impressive, must answer the question: Is it paying off?
A perfect RAG implementation doesn't just make work easier; it contributes to the bottom line. By streamlining operations, accelerating output, and even unlocking new revenue channels, EKAs can deliver measurable ROI that justifies scale and expansion.
How to Measure
- Cost Savings:
- Time saved by employees - Translate hours saved into full-time equivalent (FTE) cost savings.
- Reduction in manual errors or rework - estimate cost avoidance.
- Decrease in onboarding/training hours - calculate associated cost reductions.
- Revenue Uplift:
- Improved customer experience → track retention, upsell rates, and NPS-linked growth.
- Faster service/project delivery → attribute revenue acceleration.
- Support more customers with fewer resources → measure throughput without increasing headcount.
RAG-Specific Considerations
RAG helps lower costs by reducing the need for constant model retraining. You retrieve knowledge in real time instead of baking it into the model. That means better scalability with fewer engineering cycles. When it's surfacing precise information, agents and reps can resolve queries faster, turning more leads into loyal customers.
Case Study
A global telecom provider rolled out a RAG-powered knowledge assistant across its support and field service teams. Within the first six months:
- It saved over 15,000 agent hours, translating to huge support cost savings.
- First-time resolution improvements and reduced churn led to a 3.8% boost in customer retention, directly tied to a great gain in annualized revenue uplift.
- Project implementation timelines for enterprise clients were shortened by 11%, allowing the company to accelerate billing cycles and onboard more clients per quarter.
Even beyond these numbers, leadership reported faster internal decision-making and reduced dependency on tribal knowledge, both of which fed back into smarter, more profitable operations.
Related Read: RAG-Powered Financial Intelligence Assistant
Conclusion
Enterprise Knowledge Assistants are no longer just intelligent add-ons; they're becoming central to how organizations access knowledge, scale support, and unlock productivity. But just as important as deploying an EKA is knowing whether it's actually making a difference.
When powered by Retrieval-Augmented Generation, the assistant goes a step further. It doesn't just find data; it delivers context-aware, citation-backed insights pulled in real time from your organization's living knowledge ecosystem. That's what turns a helpful tool into a competitive advantage.
At Signity, we specialize in building customized, enterprise-level, RAG-powered Knowledge Assistants tailored to your industry, workflows, and knowledge infrastructure. From architecture to deployment to continuous optimization, our team ensures your assistant doesn't just function; it thrives, adapts, and scales.
If you're looking for RAG development services, connect with us to explore how RAG can transform how your teams think, act, and deliver.
Frequently Asked Questions
Have a question in mind? We are here to answer. If you don’t see your question here, drop us a line at our contact page.
What is an enterprise knowledge assistant?
An enterprise knowledge assistant helps employees quickly find answers, documents, or policies without digging through endless folders or bugging teammates. It sits inside tools you already use, like Slack or Teams, and responds to questions in plain language by pulling accurate, relevant info from your internal systems. It's like ChatGPT but trained on your company's knowledge.
Why should we even bother measuring the ROI of a knowledge assistant?
How does RAG make a knowledge assistant better?
What should we actually track to know if the assistant is doing its job?
To see if your knowledge assistant is truly working, focus on the impact it has on everyday operations. Are employees finding answers faster? Are new hires getting up to speed without heavy handholding? Is customer support more efficient? And is all of this leading to real cost savings or revenue gains? If it's making work smoother and measurable results are showing, you're on the right track.